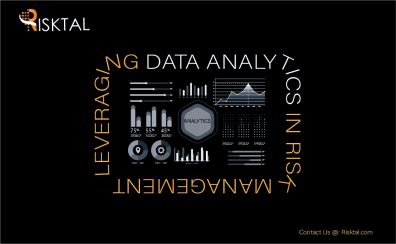
Leveraging Data Analytics in Risk Management
In an increasingly complex business environment, the ability to anticipate and mitigate risks is more important than ever. Traditionally, risk management has relied on historical data and manual processes to identify potential threats. However, the advent of data analytics and artificial intelligence (AI) is transforming how organizations approach risk management. By leveraging these advanced technologies, companies can gain deeper insights into potential risks, predict future challenges, and respond more effectively to emerging threats.
The Role of Data Analytics in Risk Management
Data analytics involves the use of statistical techniques, algorithms, and software tools to analyze large datasets and extract meaningful patterns. In risk management, data analytics can help organizations identify and quantify risks that might otherwise go unnoticed. By analyzing historical data, companies can uncover trends and correlations that can inform decision-making and improve risk mitigation strategies.
Key benefits of using data analytics in risk management include:
- Improved Risk Identification: Traditional risk management methods often rely on intuition and experience, which can lead to incomplete or biased risk assessments. Data analytics, on the other hand, provides a more objective approach by analyzing vast amounts of data from various sources. This enables organizations to identify risks more accurately and comprehensively.
- Predictive Analytics: One of the most powerful applications of data analytics in risk management is predictive analytics. By using machine learning algorithms, organizations can predict future risks based on historical data. For example, predictive models can forecast potential supply chain disruptions, financial losses, or cybersecurity threats, allowing companies to take proactive measures.
- Real-Time Risk Monitoring: Data analytics tools can continuously monitor risk indicators in real-time, providing organizations with timely alerts when certain thresholds are exceeded. This enables a more agile response to emerging risks, minimizing potential impacts on the business.
Applications of AI and Machine Learning in Risk Management
AI and machine learning are key components of data analytics that can further enhance risk management capabilities. These technologies can process vast amounts of data much faster than humans and identify patterns that may not be immediately apparent.
Some applications include:
- Fraud Detection: Machine learning algorithms can analyze transactional data to detect anomalies that may indicate fraudulent activity. By learning from historical fraud patterns, these algorithms can flag suspicious transactions in real-time, helping organizations prevent financial losses.
- Cybersecurity: AI-driven tools can detect and respond to cybersecurity threats by analyzing network traffic, user behavior, and other data points. These tools can identify potential security breaches before they occur and take corrective action automatically, reducing the risk of data breaches.
- Supply Chain Risk Management: Machine learning models can predict potential disruptions in the supply chain by analyzing data from various sources, such as weather reports, geopolitical events, and supplier performance metrics. This allows companies to mitigate risks by adjusting their supply chain strategies in advance.
Challenges and Considerations
While data analytics and AI offer significant advantages in risk management, there are also challenges to consider:
- Data Quality: The effectiveness of data analytics depends on the quality of the data being analyzed. Inaccurate or incomplete data can lead to incorrect conclusions and ineffective risk management strategies. Organizations must ensure that their data is accurate, consistent, and up-to-date.
- Integration with Existing Systems: Implementing data analytics tools requires integration with existing risk management systems and processes. This can be complex and may require significant investment in technology and training.
- Privacy and Ethical Concerns: The use of data analytics and AI in risk management raises concerns about privacy and ethics. Organizations must ensure that they comply with data protection regulations and use data responsibly.
In Summary
Data analytics and AI are revolutionizing the field of risk management by providing organizations with deeper insights, predictive capabilities, and real-time monitoring. By leveraging these technologies, companies can identify risks more accurately, predict future challenges, and respond more effectively to emerging threats. However, to fully realize the benefits, organizations must address challenges related to data quality, system integration, and ethical considerations. As these technologies continue to evolve, they will play an increasingly critical role in helping businesses navigate the complexities of the modern risk landscape.